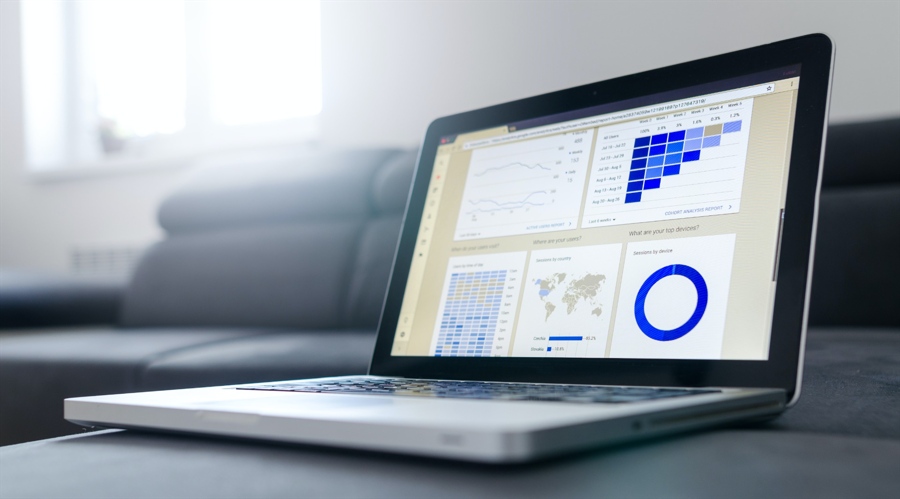
Credit scoring
is undergoing a transition as a result of technological and data analytics
improvements. While traditional credit scoring models are useful, they have
drawbacks that are being addressed through novel approaches. New technologies
are altering how creditworthiness is assessed, from different data sources to
machine learning algorithms.
We look at the
most recent advances in credit scoring, their potential benefits, and the
changing lending landscape.
Traditional
Credit Scoring Issues
Traditional
credit score models largely consider payment history, credit utilization,
credit history length, credit kinds, and current credit queries. While these
models have shown to be viable tools for determining creditworthiness, they do
have some limitations:
- Individuals
with weak credit histories or those without access to typical financial
institutions may be excluded from traditional credit rating models. - Lack of
Context: These models may fail to capture an individual’s entire financial
profile, neglecting aspects that could provide a more comprehensive picture of
creditworthiness. - Slow
Adaptation: Traditional models may have difficulty fast adapting to shifting
financial practices or unorthodox financing conditions.
The
Importance of Alternative Data
The inclusion
of other data sources is one of the most significant changes in credit rating.
Non-traditional financial data comprises information about an individual’s
financial activity that goes beyond what typical models consider. Alternative
data may include:
- Utility and
rent payments: Ongoing utility and rent payments can reflect financial
responsibility and are now taken into account when calculating credit scores. - Digital
Footprints: Creditworthiness is being determined by analyzing online behavior
such as social media activity and online buying patterns. - Education and
Work Experience: Some models regard educational and work experience as
predictors of stability and future earning potential.
Predictive
Analytics and Machine Learning
Machine
learning algorithms are transforming credit scoring by analyzing massive
volumes of data to uncover patterns and connections that traditional models may
miss. These algorithms are constantly learning and adapting, increasing their
accuracy over time.
They are able
to:
- Identify
Complex linkages: Machine learning can reveal complex linkages between
variables that affect creditworthiness. - Personalize
Scoring: Algorithms can generate personalized credit profiles based on an
individual’s financial habits and circumstances. - Machine
learning algorithms can forecast future credit behaviors and assess risk more
effectively by studying previous data.
Identity
Verification and Blockchain
Through
improved identity verification and data protection, blockchain technology is
also making inroads into credit scoring. Blockchain:
- Ensures Data
Integrity: Once data is recorded on the blockchain, it cannot be changed,
creating a tamper-proof record of a person’s financial history. - Individuals
have control over their personal data, which allows them to share only relevant
information with lenders. - Reduces Fraud:
The transparency and security measures of blockchain can aid in the reduction
of identity fraud and the protection of sensitive information.
Open Banking
and User-Generated Data
Individuals can
share their financial data with authorized third parties thanks to the open
banking movement. This allows lenders to access real-time financial data,
providing them with a more up-to-date picture of an individual’s financial
status. It also empowers customers by giving them more control over their
financial data.
Considerations
and Benefits
- Credit Access
for the Underserved: Alternative data and creative scoring methods can open up
credit to those who were previously denied owing to a lack of credit history. - More Accurate
Evaluations: New methodologies provide a more detailed view of a person’s
creditworthiness, potentially lowering instances of over- or under-lending. - Fairness &
Bias Mitigation: By relying on alternative data that presents a more
diversified picture of financial behavior, some models try to moderate biases
that standard models may perpetuate. - Concerns about
data privacy and security arise from the incorporation of alternative data. It
is critical to find a balance between information availability and the
protection of people’ sensitive data. - Considerations
for Regulatory authorities: As credit scoring models evolve, regulatory
authorities must adapt to guarantee that new techniques comply with consumer
protection rules.
Generational Trends in
Credit Card Debt: Gen Z Rising, Gen X Leading
Recent data from
Credit Karma reveals shifting patterns in credit card debt across
generations. During Q2 2023, Gen Z (born 1997-2012) saw their average credit
card balance increase to $3,328, a 4.23% jump from the previous quarter when it
stood at $3,193. This rise could be attributed to increased spending on
electronics, computers, and streaming services during the pandemic. Dr.
Balbinder Singh Gill, an assistant professor of finance at the School of
Business at Stevens Institute of Technology, suggests this.
The total credit card balances
for Americans hit
a record $1 trillion in 2023, with a $45 billion increase in Q2 alone,
marking over a 4% uptick from the prior quarter. This surge contributed
significantly to the total household debt, reaching $17.6 trillion in Q2 2023.
The Baby Boomers (born 1946-1964) hold the second-highest credit card debt,
averaging about $8,192, as per Credit Karma.
Gen X (born 1965-1980) carries
the highest average credit card balance, recording $9,589 between April and
June, a 1.89% increase from the previous quarter. Older generations like Baby
Boomers and the Silent Generation are spending more on leisure activities, with
Gen X at the pinnacle of their careers, leading to increased earnings and an
appetite for major purchases, including homes and cars.
Millennials (born 1981-1996)
witnessed the second-highest increase in credit card debt in Q2 at 2.55%,
holding an average debt of $6,959. Their spending habits often revolve around
hobbies, clothing, electronics, and socializing.
Conclusion
The expanding
landscape of credit scoring is characterized by game-changing technologies that
have the potential to change lending and financial inclusion. Alternative data,
machine learning, blockchain, open banking, and data contributed by consumers
are forging a future in which credit assessments are more accurate, tailored,
and fair.
However, as the
sector embraces new advances, ethical considerations, data privacy, and
regulatory alignment will become increasingly important in ensuring that these
advancements benefit both lenders and borrowers. As the financial services
industry embraces these improvements, it will create a more inclusive and
dynamic credit ecosystem.
Credit scoring
is undergoing a transition as a result of technological and data analytics
improvements. While traditional credit scoring models are useful, they have
drawbacks that are being addressed through novel approaches. New technologies
are altering how creditworthiness is assessed, from different data sources to
machine learning algorithms.
We look at the
most recent advances in credit scoring, their potential benefits, and the
changing lending landscape.
Traditional
Credit Scoring Issues
Traditional
credit score models largely consider payment history, credit utilization,
credit history length, credit kinds, and current credit queries. While these
models have shown to be viable tools for determining creditworthiness, they do
have some limitations:
- Individuals
with weak credit histories or those without access to typical financial
institutions may be excluded from traditional credit rating models. - Lack of
Context: These models may fail to capture an individual’s entire financial
profile, neglecting aspects that could provide a more comprehensive picture of
creditworthiness. - Slow
Adaptation: Traditional models may have difficulty fast adapting to shifting
financial practices or unorthodox financing conditions.
The
Importance of Alternative Data
The inclusion
of other data sources is one of the most significant changes in credit rating.
Non-traditional financial data comprises information about an individual’s
financial activity that goes beyond what typical models consider. Alternative
data may include:
- Utility and
rent payments: Ongoing utility and rent payments can reflect financial
responsibility and are now taken into account when calculating credit scores. - Digital
Footprints: Creditworthiness is being determined by analyzing online behavior
such as social media activity and online buying patterns. - Education and
Work Experience: Some models regard educational and work experience as
predictors of stability and future earning potential.
Predictive
Analytics and Machine Learning
Machine
learning algorithms are transforming credit scoring by analyzing massive
volumes of data to uncover patterns and connections that traditional models may
miss. These algorithms are constantly learning and adapting, increasing their
accuracy over time.
They are able
to:
- Identify
Complex linkages: Machine learning can reveal complex linkages between
variables that affect creditworthiness. - Personalize
Scoring: Algorithms can generate personalized credit profiles based on an
individual’s financial habits and circumstances. - Machine
learning algorithms can forecast future credit behaviors and assess risk more
effectively by studying previous data.
Identity
Verification and Blockchain
Through
improved identity verification and data protection, blockchain technology is
also making inroads into credit scoring. Blockchain:
- Ensures Data
Integrity: Once data is recorded on the blockchain, it cannot be changed,
creating a tamper-proof record of a person’s financial history. - Individuals
have control over their personal data, which allows them to share only relevant
information with lenders. - Reduces Fraud:
The transparency and security measures of blockchain can aid in the reduction
of identity fraud and the protection of sensitive information.
Open Banking
and User-Generated Data
Individuals can
share their financial data with authorized third parties thanks to the open
banking movement. This allows lenders to access real-time financial data,
providing them with a more up-to-date picture of an individual’s financial
status. It also empowers customers by giving them more control over their
financial data.
Considerations
and Benefits
- Credit Access
for the Underserved: Alternative data and creative scoring methods can open up
credit to those who were previously denied owing to a lack of credit history. - More Accurate
Evaluations: New methodologies provide a more detailed view of a person’s
creditworthiness, potentially lowering instances of over- or under-lending. - Fairness &
Bias Mitigation: By relying on alternative data that presents a more
diversified picture of financial behavior, some models try to moderate biases
that standard models may perpetuate. - Concerns about
data privacy and security arise from the incorporation of alternative data. It
is critical to find a balance between information availability and the
protection of people’ sensitive data. - Considerations
for Regulatory authorities: As credit scoring models evolve, regulatory
authorities must adapt to guarantee that new techniques comply with consumer
protection rules.
Generational Trends in
Credit Card Debt: Gen Z Rising, Gen X Leading
Recent data from
Credit Karma reveals shifting patterns in credit card debt across
generations. During Q2 2023, Gen Z (born 1997-2012) saw their average credit
card balance increase to $3,328, a 4.23% jump from the previous quarter when it
stood at $3,193. This rise could be attributed to increased spending on
electronics, computers, and streaming services during the pandemic. Dr.
Balbinder Singh Gill, an assistant professor of finance at the School of
Business at Stevens Institute of Technology, suggests this.
The total credit card balances
for Americans hit
a record $1 trillion in 2023, with a $45 billion increase in Q2 alone,
marking over a 4% uptick from the prior quarter. This surge contributed
significantly to the total household debt, reaching $17.6 trillion in Q2 2023.
The Baby Boomers (born 1946-1964) hold the second-highest credit card debt,
averaging about $8,192, as per Credit Karma.
Gen X (born 1965-1980) carries
the highest average credit card balance, recording $9,589 between April and
June, a 1.89% increase from the previous quarter. Older generations like Baby
Boomers and the Silent Generation are spending more on leisure activities, with
Gen X at the pinnacle of their careers, leading to increased earnings and an
appetite for major purchases, including homes and cars.
Millennials (born 1981-1996)
witnessed the second-highest increase in credit card debt in Q2 at 2.55%,
holding an average debt of $6,959. Their spending habits often revolve around
hobbies, clothing, electronics, and socializing.
Conclusion
The expanding
landscape of credit scoring is characterized by game-changing technologies that
have the potential to change lending and financial inclusion. Alternative data,
machine learning, blockchain, open banking, and data contributed by consumers
are forging a future in which credit assessments are more accurate, tailored,
and fair.
However, as the
sector embraces new advances, ethical considerations, data privacy, and
regulatory alignment will become increasingly important in ensuring that these
advancements benefit both lenders and borrowers. As the financial services
industry embraces these improvements, it will create a more inclusive and
dynamic credit ecosystem.
- SEO Powered Content & PR Distribution. Get Amplified Today.
- PlatoData.Network Vertical Generative Ai. Empower Yourself. Access Here.
- PlatoAiStream. Web3 Intelligence. Knowledge Amplified. Access Here.
- PlatoESG. Automotive / EVs, Carbon, CleanTech, Energy, Environment, Solar, Waste Management. Access Here.
- PlatoHealth. Biotech and Clinical Trials Intelligence. Access Here.
- ChartPrime. Elevate your Trading Game with ChartPrime. Access Here.
- BlockOffsets. Modernizing Environmental Offset Ownership. Access Here.
- Source: https://www.financemagnates.com//trending/upgrading-credit-scoring-unveiling-the-latest-innovations/