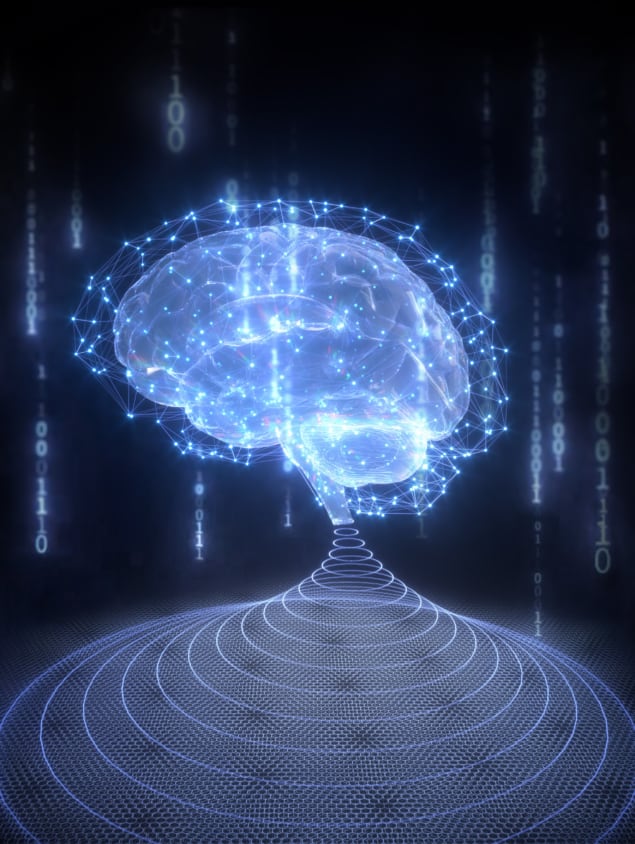
Researchers at Northwestern University, Boston College and the Massachusetts Institute of Technology (MIT), all in the US, have developed a new type of transistor for use in neuromorphic computing. The device, which works at room temperatures, can be trained to recognize similar patterns of inputs – a property known as associative learning that goes beyond standard machine-learning tasks.
Neuromorphic computers, as their name suggests, are inspired by the architecture of the human brain. The building blocks of their circuits are highly connected artificial neurons and artificial synapses that simulate the brain’s structure and functions. These machines have combined processing and memory units that allow them to process information at the same time as they store it – just like a multi-tasking human brain. This ability sets them apart from digital computers with separate processing and storage units, which consume huge amounts of energy when performing data-intensive tasks. Such tasks are becoming increasingly commonplace with the arrival of smart, connected devices and vast datasets.
While synaptic devices have progressed significantly in recent years, they are limited by a lack of good switching mechanisms, explains Mark Hersam of Northwestern, who co-led the research effort. “The stochastic nature of filamentary switching in memristors (short for memory resistors), which are the most common synaptic technology today, leads to significant device-to-device and cycle-to-cycle variability,” he says.
Other types of synaptic devices rely on magnetic and phase change switching, but these suffer from low switching ratios and high switching energies, respectively, Hersam adds.
Moiré quantum materials
To overcome these problems, Hersam and colleagues have been studying two-dimensional moiré quantum materials. These are made up of layers of different atomically thin materials stacked atop each other and twisted by small angles. Such structures have electronic properties that do not exist in individual layers of material. By twisting the layers at different angles relative to each other, researchers can tune these electronic properties very precisely – a property that is very attractive for new electronic devices, including components for neuromorphic computing.
In their work, which is detailed in Nature, the researchers created an asymmetric structure made up of two layers of graphene (a flat crystal of carbon just one atom thick) and a layer of hexagonal boron nitride (hBN). Since these two materials have very similar lattice constants, the moiré effects caused by the slight mismatch in the locations of their atoms are very pronounced. The result is a strong Coulomb coupling between the bipartite electronic states in the heterostructure that manifests itself as an electronically controlled ratcheting mechanism. This ratchet allows the conductance of a transistor made from the heterostructure to be precisely controlled and continually tuned.
“The continuous tuneability of the device conductance yields dense and programmable memory states in addition to novel quantum synaptic functions, such as bio-realistic homeostasis and input-specific adaptation,” explains Hersam. “What is more, our devices consume very little power and show minimal device-to-device variations thanks to the homogeneity of the moiré electronic states.”
Room temperature operation
And that is not all: the devices switch quickly, retain their electronic states even when the power is switched off and, importantly, are stable at room temperature. This is in contrast to previous moiré devices that only functioned at cryogenic temperatures.
To test their transistor, Hersam and team trained it to recognize patterns that look similar to each other. They began by inputting a sequence of three zeros in a row (000) and then tested it to identify similar patterns, such as 111 or 101.
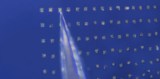
Memristors make versatile artificial synapses for neuromorphic computing
“If we trained it to detect 000 and then gave it 111 and 101, it knows 111 is more similar to 000 than 101,” explains Hersam. “000 and 111 are not exactly the same, but both are three digits in a row.”
Recognizing similarity is a higher-level form of cognition known as associative learning and the new device is capable of this, he says.
The researchers are now exploring the potential of other van der Waals materials beyond graphene and hBN, hoping to integrate them into moiré heterostructures with even more sophisticated neuromorphic functionality. “A longer-term goal would be to scale up the most promising examples among these heterostructures to realize fully integrated neuromorphic circuits and systems,” Hersam tells Physics World.
- SEO Powered Content & PR Distribution. Get Amplified Today.
- PlatoData.Network Vertical Generative Ai. Empower Yourself. Access Here.
- PlatoAiStream. Web3 Intelligence. Knowledge Amplified. Access Here.
- PlatoESG. Carbon, CleanTech, Energy, Environment, Solar, Waste Management. Access Here.
- PlatoHealth. Biotech and Clinical Trials Intelligence. Access Here.
- Source: https://physicsworld.com/a/moire-material-makes-a-synaptic-transistor-for-neuromorphic-computing/