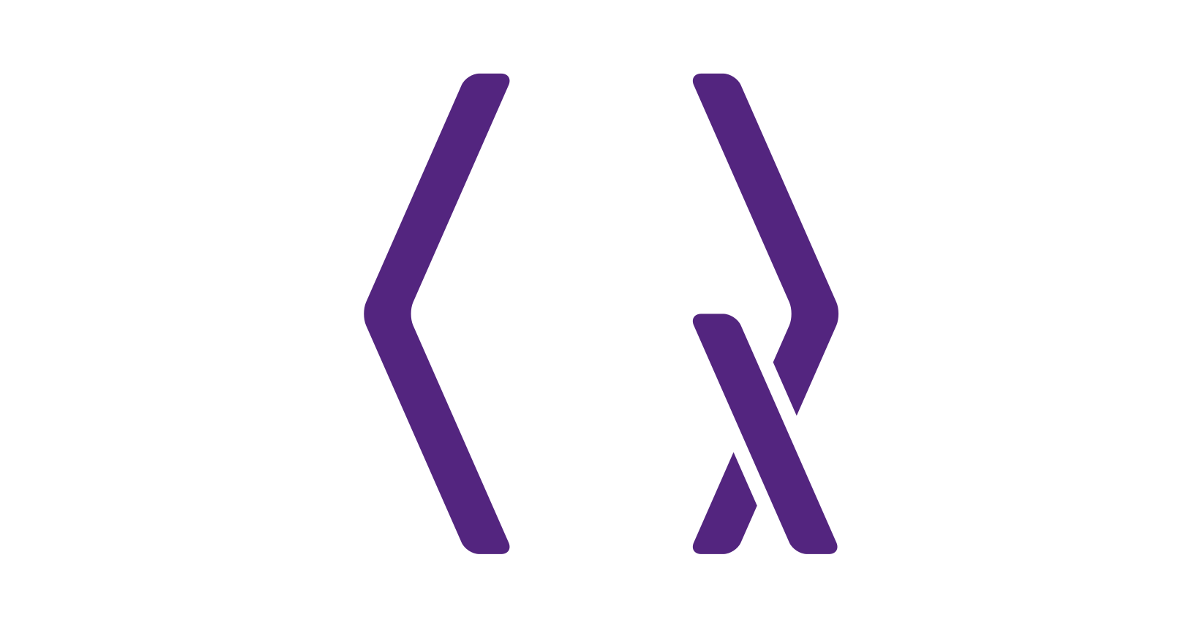
1Department of Mathematics, Technische Universität München, 85748 Garching, Germany
2QMATH, Department of Mathematical Sciences, University of Copenhagen, Denmark
3Univ Lyon, ENS Lyon, UCBL, CNRS, Inria, LIP, F-69342, Lyon Cedex 07, France
Find this paper interesting or want to discuss? Scite or leave a comment on SciRate.
Abstract
Estimating physical properties of quantum states from measurements is one of the most fundamental tasks in quantum science. In this work, we identify conditions on states under which it is possible to infer the expectation values of all quasi-local observables of a state from a number of copies that scales polylogarithmically with the system’s size and polynomially on the locality of the target observables. We show that this constitutes a provable exponential improvement in the number of copies over state-of-the-art tomography protocols. We achieve our results by combining the maximum entropy method with tools from the emerging fields of classical shadows and quantum optimal transport. The latter allows us to fine-tune the error made in estimating the expectation value of an observable in terms of how local it is and how well we approximate the expectation value of a fixed set of few-body observables. We conjecture that our condition holds for all states exhibiting some form of decay of correlations and establish it for several subsets thereof. These include widely studied classes of states such as one-dimensional thermal and high-temperature Gibbs states of local commuting Hamiltonians on arbitrary hypergraphs or outputs of shallow circuits. Moreover, we show improvements of the maximum entropy method beyond the sample complexity that are of independent interest. These include identifying regimes in which it is possible to perform the postprocessing efficiently as well as novel bounds on the condition number of covariance matrices of many-body states.
[embedded content]
Popular summary
The new approach introduced in this work harnesses the power of maximum entropy methods combined with techniques from classical shadows and quantum optimal transport. Specifically, the new protocol can infer the values of all quasi-local observables of a state from a dramatically reduced number of copies that scale polylogarithmically with the system’s size. This constitutes a major advancement over previous state-of-the-art tomography protocols, which often required a dauntingly large number of samples.
In practical terms, this means that scientists can now measure and predict the behavior of certain quantum systems with fewer resources.
► BibTeX data
► References
[1] Scott Aaronson. Shadow tomography of quantum states. In STOC’18—Proceedings of the 50th Annual ACM SIGACT Symposium on Theory of Computing, pages 325–338. ACM, New York, 2018. 10.1145/3188745.3188802.
https://doi.org/10.1145/3188745.3188802
[2] Álvaro M. Alhambra and J. Ignacio Cirac. Locally accurate tensor networks for thermal states and time evolution. PRX Quantum, 2 (4), November 2021. ISSN 2691-3399. 10.1103/prxquantum.2.040331.
https://doi.org/10.1103/prxquantum.2.040331
[3] Anurag Anshu. Concentration bounds for quantum states with finite correlation length on quantum spin lattice systems. New Journal of Physics, 18 (8): 083011, aug 2016. 10.1088/1367-2630/18/8/083011.
https://doi.org/10.1088/1367-2630/18/8/083011
[4] Anurag Anshu, Srinivasan Arunachalam, Tomotaka Kuwahara, and Mehdi Soleimanifar. Efficient learning of commuting hamiltonians on lattices. Unpublished notes avaible at Anurag Anshu’s website, (link to note).
https://people.eecs.berkeley.edu/~anuraganshu/Learning_commuting_hamiltonian.pdf
[5] Anurag Anshu, Srinivasan Arunachalam, Tomotaka Kuwahara, and Mehdi Soleimanifar. Sample-efficient learning of interacting quantum systems. Nature Physics, may 2021. ISSN 1745-2473. 10.1038/s41567-021-01232-0.
https://doi.org/10.1038/s41567-021-01232-0
[6] Huzihiro Araki. Gibbs states of a one dimensional quantum lattice. Communications in Mathematical Physics, 14 (2): 120–157, 1969. 10.1007/bf01645134.
https://doi.org/10.1007/bf01645134
[7] Ivan Bardet, Angela Capel, and Cambyse Rouzé. Approximate tensorization of the relative entropy for noncommuting conditional expectations. arXiv preprint arXiv:2001.07981, 2020. 10.1007/s00023-021-01088-3.
https://doi.org/10.1007/s00023-021-01088-3
arXiv:2001.07981
[8] Ivan Bardet, Ángela Capel, and Cambyse Rouzé. Approximate tensorization of the relative entropy for noncommuting conditional expectations. Annales Henri Poincaré, 23 (1): 101–140, July 2021. ISSN 1424-0661. 10.1007/s00023-021-01088-3.
https://doi.org/10.1007/s00023-021-01088-3
[9] Thomas Barthel and Martin Kliesch. Quasilocality and efficient simulation of markovian quantum dynamics. Physical review letters, 108 (23): 230504, 2012. 10.1103/physrevlett.108.230504.
https://doi.org/10.1103/physrevlett.108.230504
[10] T Baumgratz, A Nüßeler, M Cramer, and M B Plenio. A scalable maximum likelihood method for quantum state tomography. New Journal of Physics, 15 (12): 125004, dec 2013. 10.1088/1367-2630/15/12/125004.
https://doi.org/10.1088/1367-2630/15/12/125004
[11] Salman Beigi, Nilanjana Datta, and Cambyse Rouzé. Quantum reverse hypercontractivity: its tensorization and application to strong converses. Communications in Mathematical Physics, 376 (2): 753–794, 2020. 10.1007/s00220-020-03750-z.
https://doi.org/10.1007/s00220-020-03750-z
[12] S.G Bobkov and F Götze. Exponential Integrability and Transportation Cost Related to Logarithmic Sobolev Inequalities. Journal of Functional Analysis, 163 (1): 1–28, April 1999. ISSN 00221236. 10.1006/jfan.1998.3326.
https://doi.org/10.1006/jfan.1998.3326
[13] Xavier Bonet-Monroig, Ryan Babbush, and Thomas E O’Brien. Nearly optimal measurement scheduling for partial tomography of quantum states. Physical Review X, 10 (3): 031064, 2020. 10.1103/physrevx.10.031064.
https://doi.org/10.1103/physrevx.10.031064
[14] Stephen Boyd, Stephen P Boyd, and Lieven Vandenberghe. Convex optimization. Cambridge university press, 2004.
[15] Fernando G. S. L. Brandao and Marcus Cramer. Equivalence of Statistical Mechanical Ensembles for Non-Critical Quantum Systems. arXiv:1502.03263 [cond-mat, physics:quant-ph], February 2015. arXiv: 1502.03263.
arXiv:1502.03263
[16] Fernando G. S. L. Brandão, Amir Kalev, Tongyang Li, Cedric Yen-Yu Lin, Krysta M. Svore, and Xiaodi Wu. Quantum SDP solvers: large speed-ups, optimality, and applications to quantum learning. In 46th International Colloquium on Automata, Languages, and Programming, volume 132 of LIPIcs. Leibniz Int. Proc. Inform., pages Art. No. 27, 14. Schloss Dagstuhl. Leibniz-Zent. Inform., Wadern, 2019.
[17] Fernando G. S. L. Brandão and Michael J. Kastoryano. Finite Correlation Length Implies Efficient Preparation of Quantum Thermal States. Communications in Mathematical Physics, 365 (1): 1–16, January 2019. ISSN 0010-3616, 1432-0916. 10.1007/s00220-018-3150-8.
https://doi.org/10.1007/s00220-018-3150-8
[18] Ángela Capel, Cambyse Rouzé, and Daniel Stilck França. The modified logarithmic sobolev inequality for quantum spin systems: classical and commuting nearest neighbour interactions. arXiv preprint arXiv:2009.11817, 2020.
arXiv:2009.11817
[19] Eric A Carlen and Jan Maas. An analog of the 2-wasserstein metric in non-commutative probability under which the fermionic fokker–planck equation is gradient flow for the entropy. Communications in mathematical physics, 331 (3): 887–926, 2014. 10.1007/s00220-014-2124-8.
https://doi.org/10.1007/s00220-014-2124-8
[20] Eric A Carlen and Jan Maas. Gradient flow and entropy inequalities for quantum Markov semigroups with detailed balance. Journal of Functional Analysis, 273 (5): 1810–1869, 2017. 10.1016/j.jfa.2017.05.003.
https://doi.org/10.1016/j.jfa.2017.05.003
[21] Eric A. Carlen and Jan Maas. Non-commutative calculus, optimal transport and functional inequalities in dissipative quantum systems. Journal of Statistical Physics, 178 (2): 319–378, nov 2019. 10.1007/s10955-019-02434-w.
https://doi.org/10.1007/s10955-019-02434-w
[22] Jordan Cotler and Frank Wilczek. Quantum overlapping tomography. Physical Review Letters, 124 (10): 100401, 2020. 10.32657/10356/170876.
https://doi.org/10.32657/10356/170876
[23] Marcus Cramer, Martin B. Plenio, Steven T. Flammia, Rolando Somma, David Gross, Stephen D. Bartlett, Olivier Landon-Cardinal, David Poulin, and Yi-Kai Liu. Efficient quantum state tomography. Nature Communications, 1 (1): 149, dec 2010. ISSN 2041-1723. 10.1038/ncomms1147.
https://doi.org/10.1038/ncomms1147
[24] Ophelia Crawford, Barnaby van Straaten, Daochen Wang, Thomas Parks, Earl Campbell, and Stephen Brierley. Efficient quantum measurement of pauli operators in the presence of finite sampling error. Quantum, 5: 385, January 2021. ISSN 2521-327X. 10.22331/q-2021-01-20-385. URL http://dx.doi.org/10.22331/q-2021-01-20-385.
https://doi.org/10.22331/q-2021-01-20-385
[25] Alexander M. Dalzell and Fernando G. S. L. Brandão. Locally accurate MPS approximations for ground states of one-dimensional gapped local Hamiltonians. Quantum, 3: 187, September 2019. ISSN 2521-327X. 10.22331/q-2019-09-23-187.
https://doi.org/10.22331/q-2019-09-23-187
[26] Giacomo De Palma and Cambyse Rouzé. Quantum concentration inequalities. Annales Henri Poincaré, 23 (9): 3391–3429, April 2022. ISSN 1424-0661. 10.1007/s00023-022-01181-1. URL http://dx.doi.org/10.1007/s00023-022-01181-1.
https://doi.org/10.1007/s00023-022-01181-1
[27] Luc Devroye, Abbas Mehrabian, and Tommy Reddad. The minimax learning rates of normal and Ising undirected graphical models. Electronic Journal of Statistics, 14 (1), January 2020. 10.1214/20-ejs1721.
https://doi.org/10.1214/20-ejs1721
[28] Roland L Dobrushin and Senya B Shlosman. Completely analytical interactions: constructive description. Journal of Statistical Physics, 46 (5): 983–1014, 1987. 10.1007/bf01011153.
https://doi.org/10.1007/bf01011153
[29] Jens Eisert, Dominik Hangleiter, Nathan Walk, Ingo Roth, Damian Markham, Rhea Parekh, Ulysse Chabaud, and Elham Kashefi. Quantum certification and benchmarking. Nature Reviews Physics, 2 (7): 382–390, jul 2020. ISSN 2522-5820. 10.1038/s42254-020-0186-4.
https://doi.org/10.1038/s42254-020-0186-4
[30] Daniel Stilck França, Fernando G.S L. Brandão, and Richard Kueng. Fast and robust quantum state tomography from few basis measurements. Schloss Dagstuhl – Leibniz-Zentrum für Informatik, 2021. 10.4230/LIPICS.TQC.2021.7.
https://doi.org/10.4230/LIPICS.TQC.2021.7
[31] Li Gao, Marius Junge, and Nicholas LaRacuente. Fisher Information and Logarithmic Sobolev Inequality for Matrix-Valued Functions. Annales Henri Poincaré, 21 (11): 3409–3478, November 2020. ISSN 1424-0637, 1424-0661. 10.1007/s00023-020-00947-9.
https://doi.org/10.1007/s00023-020-00947-9
[32] Nathael Gozlan and Christian Léonard. Transport inequalities. a survey. arXiv preprint arXiv:1003.3852, 2010.
arXiv:1003.3852
[33] Jeongwan Haah, Aram Wettroth Harrow, Zhengfeng Ji, Xiaodi Wu, and Nengkun Yu. Sample-optimal tomography of quantum states. IEEE Trans. Inf. Theory, 63 (9): 5628–5641, 2017. 10.1109/TIT.2017.2719044.
https://doi.org/10.1109/TIT.2017.2719044
[34] Jeongwan Haah, Robin Kothari, and Ewin Tang. Optimal learning of quantum Hamiltonians from high-temperature Gibbs states, 2021. arXiv:2108.04842v1.
arXiv:2108.04842v1
[35] Aram W Harrow, Saeed Mehraban, and Mehdi Soleimanifar. Classical algorithms, correlation decay, and complex zeros of partition functions of quantum many-body systems. In Proceedings of the 52nd Annual ACM SIGACT Symposium on Theory of Computing, pages 378–386, 2020. 10.1145/3357713.3384322.
https://doi.org/10.1145/3357713.3384322
[36] M. B. Hastings. Quantum belief propagation: An algorithm for thermal quantum systems. Physical Review B, 76 (20), nov 2007a. 10.1103/physrevb.76.201102.
https://doi.org/10.1103/physrevb.76.201102
[37] M. B. Hastings. Quantum belief propagation: An algorithm for thermal quantum systems. Physical Review B, 76 (20): 201102, November 2007b. ISSN 1098-0121, 1550-235X. 10.1103/PhysRevB.76.201102.
https://doi.org/10.1103/PhysRevB.76.201102
[38] M. B. Hastings. Locality in Quantum Systems. arXiv:1008.5137 [math-ph, physics:quant-ph], August 2010. arXiv: 1008.5137.
arXiv:1008.5137
[39] Matthew B. Hastings and Tohru Koma. Spectral Gap and Exponential Decay of Correlations. Communications in Mathematical Physics, 265 (3): 781–804, August 2006. ISSN 0010-3616, 1432-0916. 10.1007/s00220-006-0030-4.
https://doi.org/10.1007/s00220-006-0030-4
[40] Hsin-Yuan Huang, Richard Kueng, and John Preskill. Predicting many properties of a quantum system from very few measurements. Nature Physics, June 2020. 10.1038/s41567-020-0932-7.
https://doi.org/10.1038/s41567-020-0932-7
[41] Yichen Huang. Locally accurate matrix product approximation to thermal states. Science Bulletin, 66 (24): 2456–2457, December 2021. ISSN 2095-9273. 10.1016/j.scib.2021.08.011. URL http://dx.doi.org/10.1016/j.scib.2021.08.011.
https://doi.org/10.1016/j.scib.2021.08.011
[42] E. T. Jaynes. Information Theory and Statistical Mechanics. Physical Review, 106 (4): 620–630, May 1957. ISSN 0031-899X. 10.1103/PhysRev.106.620.
https://doi.org/10.1103/PhysRev.106.620
[43] Andrew Jena, Scott Genin, and Michele Mosca. Pauli Partitioning with Respect to Gate Sets. arXiv:1907.07859 [quant-ph], July 2019. arXiv: 1907.07859.
arXiv:1907.07859
[44] Michael J. Kastoryano and Fernando G. S. L. Brandão. Quantum gibbs samplers: The commuting case. Communications in Mathematical Physics, 344 (3): 915–957, May 2016. ISSN 1432-0916. 10.1007/s00220-016-2641-8.
https://doi.org/10.1007/s00220-016-2641-8
[45] Bobak Toussi Kiani, Giacomo De Palma, Milad Marvian, Zi-Wen Liu, and Seth Lloyd. Quantum earth mover’s distance: A new approach to learning quantum data. 10.1088/2058-9565/ac79c9. arXiv preprint arXiv:2101.03037, 2021.
https://doi.org/10.1088/2058-9565/ac79c9
arXiv:2101.03037
[46] M. Kliesch, C. Gogolin, M.J. Kastoryano, A. Riera, and J. Eisert. Locality of Temperature. Physical Review X, 4 (3): 031019, July 2014a. ISSN 2160-3308. 10.1103/PhysRevX.4.031019.
https://doi.org/10.1103/PhysRevX.4.031019
[47] Martin Kliesch, Christian Gogolin, and Jens Eisert. Lieb-Robinson Bounds and the Simulation of Time-Evolution of Local Observables in Lattice Systems. In Volker Bach and Luigi Delle Site, editors, Many-Electron Approaches in Physics, Chemistry and Mathematics, pages 301–318. Springer International Publishing, Cham, 2014b. ISBN 978-3-319-06378-2 978-3-319-06379-9. 10.1007/978-3-319-06379-9_17. Series Title: Mathematical Physics Studies.
https://doi.org/10.1007/978-3-319-06379-9_17
[48] Christian Kokail, Rick van Bijnen, Andreas Elben, Benoît Vermersch, and Peter Zoller. Entanglement hamiltonian tomography in quantum simulation. Nature Physics, 17 (8): 936–942, June 2021. ISSN 1745-2481. 10.1038/s41567-021-01260-w.
https://doi.org/10.1038/s41567-021-01260-w
[49] Tomotaka Kuwahara and Keiji Saito. Gaussian concentration bound and ensemble equivalence in generic quantum many-body systems including long-range interactions. Annals of Physics, 421: 168278, 2020a. 10.1016/j.aop.2020.168278.
https://doi.org/10.1016/j.aop.2020.168278
[50] Tomotaka Kuwahara and Keiji Saito. Eigenstate Thermalization from the Clustering Property of Correlation. Physical Review Letters, 124 (20): 200604, May 2020b. ISSN 0031-9007, 1079-7114. 10.1103/PhysRevLett.124.200604.
https://doi.org/10.1103/PhysRevLett.124.200604
[51] Tomotaka Kuwahara, Kohtaro Kato, and Fernando G. S. L. Brandão. Clustering of conditional mutual information for quantum Gibbs states above a threshold temperature. Phys. Rev. Lett., 124: 220601, Jun 2020. 10.1103/PhysRevLett.124.220601.
https://doi.org/10.1103/PhysRevLett.124.220601
[52] Tomotaka Kuwahara, Álvaro M. Alhambra, and Anurag Anshu. Improved Thermal Area Law and Quasilinear Time Algorithm for Quantum Gibbs States. Physical Review X, 11 (1): 011047, March 2021. ISSN 2160-3308. 10.1103/PhysRevX.11.011047.
https://doi.org/10.1103/PhysRevX.11.011047
[53] B. P. Lanyon, C. Maier, M. Holzäpfel, T. Baumgratz, C. Hempel, P. Jurcevic, I. Dhand, A. S. Buyskikh, A. J. Daley, M. Cramer, M. B. Plenio, R. Blatt, and C. F. Roos. Efficient tomography of a quantum many-body system. Nature Physics, 13 (12): 1158–1162, September 2017. 10.1038/nphys4244.
https://doi.org/10.1038/nphys4244
[54] Andrea Montanari et al. Computational implications of reducing data to sufficient statistics. Electronic Journal of Statistics, 9 (2): 2370–2390, 2015. 10.1214/15-ejs1059.
https://doi.org/10.1214/15-ejs1059
[55] Ryan O’Donnell and John Wright. Efficient quantum tomography. In Daniel Wichs and Yishay Mansour, editors, Proceedings of the 48th Annual ACM SIGACT Symposium on Theory of Computing, STOC 2016, Cambridge, MA, USA, June 18-21, 2016, pages 899–912. ACM, 2016. 10.1145/2897518.2897544.
https://doi.org/10.1145/2897518.2897544
[56] Giacomo De Palma, Milad Marvian, Dario Trevisan, and Seth Lloyd. The quantum wasserstein distance of order 1. IEEE Transactions on Information Theory, pages 1–1, 2021. 10.1109/tit.2021.3076442.
https://doi.org/10.1109/tit.2021.3076442
[57] David Poulin. Lieb-Robinson Bound and Locality for General Markovian Quantum Dynamics. Physical Review Letters, 104 (19): 190401, May 2010. ISSN 0031-9007, 1079-7114. 10.1103/PhysRevLett.104.190401.
https://doi.org/10.1103/PhysRevLett.104.190401
[58] Maxim Raginsky and Igal Sason. Concentration of Measure Inequalities in Information Theory, Communications, and Coding. Now Publishers, Norwell, MA, 2014. ISBN 978-1-60198-907-9. OCLC: 1193114287.
[59] Cambyse Rouzé and Nilanjana Datta. Concentration of quantum states from quantum functional and transportation cost inequalities. Journal of Mathematical Physics, 60 (1): 012202, January 2019. 10.1063/1.5023210.
https://doi.org/10.1063/1.5023210
[60] Brian Swingle and Isaac H. Kim. Reconstructing quantum states from local data. Physical Review Letters, 113 (26): 260501, December 2014. ISSN 0031-9007, 1079-7114. 10.1103/PhysRevLett.113.260501. arXiv: 1407.2658.
https://doi.org/10.1103/PhysRevLett.113.260501
[61] M. Talagrand. Transportation cost for Gaussian and other product measures. Geometric and Functional Analysis, 6 (3): 587–600, may 1996. 10.1007/bf02249265.
https://doi.org/10.1007/bf02249265
[62] Hal Tasaki. On the Local Equivalence Between the Canonical and the Microcanonical Ensembles for Quantum Spin Systems. Journal of Statistical Physics, 172 (4): 905–926, August 2018. ISSN 0022-4715, 1572-9613. 10.1007/s10955-018-2077-y.
https://doi.org/10.1007/s10955-018-2077-y
[63] Kristan Temme, Fernando Pastawski, and Michael J Kastoryano. Hypercontractivity of quasi-free quantum semigroups. Journal of Physics A: Mathematical and Theoretical, 47 (40): 405303, October 2014. ISSN 1751-8113, 1751-8121. 10.1088/1751-8113/47/40/405303.
https://doi.org/10.1088/1751-8113/47/40/405303
[64] Giacomo Torlai, Guglielmo Mazzola, Juan Carrasquilla, Matthias Troyer, Roger Melko, and Giuseppe Carleo. Neural-network quantum state tomography. Nature Physics, 14 (5): 447–450, may 2018. ISSN 1745-2473. 10.1038/s41567-018-0048-5.
https://doi.org/10.1038/s41567-018-0048-5
[65] Cédric Villani. Optimal Transport, volume 338 of Grundlehren der mathematischen Wissenschaften. Springer Berlin Heidelberg, Berlin, Heidelberg, 2009. ISBN 978-3-540-71049-3 978-3-540-71050-9. 10.1007/978-3-540-71050-9.
https://doi.org/10.1007/978-3-540-71050-9
[66] Marc Vuffray, Sidhant Misra, Andrey Lokhov, and Michael Chertkov. Interaction screening: Efficient and sample-optimal learning of Ising models. In Advances in Neural Information Processing Systems, pages 2595–2603, 2016.
[67] Jun Wang, Zhao-Yu Han, Song-Bo Wang, Zeyang Li, Liang-Zhu Mu, Heng Fan, and Lei Wang. Scalable quantum tomography with fidelity estimation. Phys. Rev. A, 101: 032321, Mar 2020. 10.1103/PhysRevA.101.032321.
https://doi.org/10.1103/PhysRevA.101.032321
[68] Akram Youssry, Christopher Ferrie, and Marco Tomamichel. Efficient online quantum state estimation using a matrix-exponentiated gradient method. New J. Phys., 21 (3): 033006, 2019. 10.1088/1367-2630/ab0438.
https://doi.org/10.1088/1367-2630/ab0438
Cited by
[1] Andreas Elben, Steven T. Flammia, Hsin-Yuan Huang, Richard Kueng, John Preskill, Benoît Vermersch, and Peter Zoller, “The randomized measurement toolbox”, Nature Reviews Physics 5 1, 9 (2023).
[2] Daniel Stilck França, Liubov A. Markovich, V. V. Dobrovitski, Albert H. Werner, and Johannes Borregaard, “Efficient and robust estimation of many-qubit Hamiltonians”, Nature Communications 15, 311 (2024).
[3] Ryan Levy, Di Luo, and Bryan K. Clark, “Classical shadows for quantum process tomography on near-term quantum computers”, Physical Review Research 6 1, 013029 (2024).
[4] Giacomo De Palma, Milad Marvian, Cambyse Rouzé, and Daniel Stilck França, “Limitations of Variational Quantum Algorithms: A Quantum Optimal Transport Approach”, PRX Quantum 4 1, 010309 (2023).
[5] Hsin-Yuan Huang, Yunchao Liu, Michael Broughton, Isaac Kim, Anurag Anshu, Zeph Landau, and Jarrod R. McClean, “Learning shallow quantum circuits”, arXiv:2401.10095, (2024).
[6] Álvaro M. Alhambra, “Quantum Many-Body Systems in Thermal Equilibrium”, PRX Quantum 4 4, 040201 (2023).
[7] Matthias C. Caro, “Learning Quantum Processes and Hamiltonians via the Pauli Transfer Matrix”, arXiv:2212.04471, (2022).
[8] Benoît Vermersch, Marko Ljubotina, J. Ignacio Cirac, Peter Zoller, Maksym Serbyn, and Lorenzo Piroli, “Many-body entropies and entanglement from polynomially-many local measurements”, arXiv:2311.08108, (2023).
[9] Ariel Shlosberg, Andrew J. Jena, Priyanka Mukhopadhyay, Jan F. Haase, Felix Leditzky, and Luca Dellantonio, “Adaptive estimation of quantum observables”, Quantum 7, 906 (2023).
[10] Marco Fanizza, Niklas Galke, Josep Lumbreras, Cambyse Rouzé, and Andreas Winter, “Learning finitely correlated states: stability of the spectral reconstruction”, arXiv:2312.07516, (2023).
[11] Ivan Bardet, Ángela Capel, Li Gao, Angelo Lucia, David Pérez-García, and Cambyse Rouzé, “Entropy Decay for Davies Semigroups of a One Dimensional Quantum Lattice”, Communications in Mathematical Physics 405 2, 42 (2024).
[12] Francisco Escudero Gutiérrez, “Simple algorithms to test and learn local Hamiltonians”, arXiv:2404.06282, (2024).
[13] Ke Wang and Xiantao Li, “Simulation-assisted learning of open quantum systems”, arXiv:2307.03858, (2023).
[14] Giacomo De Palma and Dario Trevisan, “The Wasserstein Distance of Order 1 for Quantum Spin Systems on Infinite Lattices”, Annales Henri Poincaré 24 12, 4237 (2023).
[15] Armando Angrisani and Elham Kashefi, “Quantum Local Differential Privacy and Quantum Statistical Query Model”, arXiv:2203.03591, (2022).
[16] Fabio Zoratti, Giacomo De Palma, Bobak Kiani, Quynh T. Nguyen, Milad Marvian, Seth Lloyd, and Vittorio Giovannetti, “Improving the speed of variational quantum algorithms for quantum error correction”, Physical Review A 108 2, 022611 (2023).
[17] Luuk Coopmans and Marcello Benedetti, “On the Sample Complexity of Quantum Boltzmann Machine Learning”, arXiv:2306.14969, (2023).
[18] Hiroshi Yano and Naoki Yamamoto, “Quantum information criteria for model selection in quantum state estimation”, Journal of Physics A Mathematical General 56 40, 405301 (2023).
[19] Da-Jian Zhang and D. M. Tong, “Inferring physical properties of symmetric states from the fewest copies”, arXiv:2301.10982, (2023).
[20] Andreas Bluhm, Matthias C. Caro, and Aadil Oufkir, “Hamiltonian Property Testing”, arXiv:2403.02968, (2024).
[21] Giacomo De Palma, Tristan Klein, and Davide Pastorello, “Classical shadows meet quantum optimal mass transport”, arXiv:2309.08426, (2023).
[22] Lennart Bittel, Antonio A. Mele, Jens Eisert, and Lorenzo Leone, “PAC-learning of free-fermionic states is NP-hard”, arXiv:2404.03585, (2024).
The above citations are from SAO/NASA ADS (last updated successfully 2024-05-02 23:46:22). The list may be incomplete as not all publishers provide suitable and complete citation data.
On Crossref’s cited-by service no data on citing works was found (last attempt 2024-05-02 23:46:21).
This Paper is published in Quantum under the Creative Commons Attribution 4.0 International (CC BY 4.0) license. Copyright remains with the original copyright holders such as the authors or their institutions.
- SEO Powered Content & PR Distribution. Get Amplified Today.
- PlatoData.Network Vertical Generative Ai. Empower Yourself. Access Here.
- PlatoAiStream. Web3 Intelligence. Knowledge Amplified. Access Here.
- PlatoESG. Carbon, CleanTech, Energy, Environment, Solar, Waste Management. Access Here.
- PlatoHealth. Biotech and Clinical Trials Intelligence. Access Here.
- Source: https://quantum-journal.org/papers/q-2024-04-30-1319/