China’s Institute of High Energy Physics (IHEP) in Beijing is pioneering innovative approaches in quantum computing and quantum machine learning to open up new research pathways within its particle physics programme, as Hideki Okawa, Weidong Li and Jun Cao explain
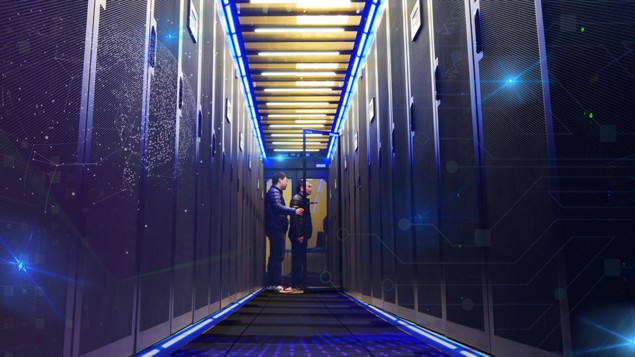
The Institute of High Energy Physics (IHEP), part of the Chinese Academy of Sciences, is the largest basic science laboratory in China. It hosts a multidisciplinary research programme spanning elementary particle physics, astrophysics as well as the planning, design and construction of large-scale accelerator projects – including the China Spallation Neutron Source, which launched in 2018, and the High Energy Photon Source, due to come online in 2025.
While investment in IHEP’s experimental infrastructure has ramped dramatically over the past 20 years, the development and application of quantum machine-learning and quantum-computing technologies is now poised to yield similarly far-reaching outcomes within the IHEP research programme.
Big science, quantum solutions
High-energy physics is where “big science” meets “big data”. Discovering new particles and probing the fundamental laws of nature are endeavours that produce incredible volumes of data. The Large Hadron Collider (LHC) at CERN generates petabytes (1015 bytes) of data during its experimental runs – all of which must be processed and analysed with the help of grid computing, a distributed infrastructure that networks computing resources worldwide.
In this way, the Worldwide LHC Computing Grid gives a community of thousands of physicists near-real-time access to LHC data. That sophisticated computing grid was fundamental to the landmark discovery of the Higgs boson at CERN in 2012 as well as countless other advances to further investigate the Standard Model of particle physics.
Another inflection point is looming, though, when it comes to the storage, analysis and mining of big data in high-energy physics. The High-Luminosity Large Hadron Collider (HL-LHC), which is anticipated to enter operation in 2029, will create a “computing crunch” as the machine’s integrated luminosity, proportional to the number of particle collisions that occur in a given amount of time, will increase by a factor of 10 versus the LHC’s design value – as will the data streams generated by the HL-LHC experiments.
CERN QTI: harnessing big science to accelerate quantum innovation
Over the near term, a new-look “computing baseline” will be needed to cope with the HL-LHC’s soaring data demands – a baseline that will require the at-scale exploitation of graphics processing units for massively parallel simulation, data recording and reprocessing, as well as classical applications of machine learning. CERN, for its part, has also established a medium- and long-term roadmap that brings together the high-energy physics and quantum technology communities via the CERN Quantum Technology Initiative (QTI) – recognition that another leap in computing performance is coming into view with the application of quantum computing and quantum networking technologies.
Back to quantum basics
Quantum computers, as the name implies, exploit the fundamental principles of quantum mechanics. Similar to classical computers, which rely on the binary bits that take the value of either 0 or 1, quantum computers exploit quantum binary bits, but as a superposition of 0 and 1 states. This superposition, coupled with quantum entanglement (correlations among quantum bits), in principle enables quantum computers to perform some types of calculation significantly faster than classical machines – for example, quantum simulations applied in various areas of quantum chemistry and molecular reaction kinetics.
While the opportunities for science and the wider economy appear compelling, one of the big engineering headaches associated with early-stage quantum computers is their vulnerability to environmental noise. Qubits are all-too-easily disturbed, for example, by their interactions with Earth’s magnetic field or stray electromagnetic fields from mobile phones and WiFi networks. Interactions with cosmic rays can also be problematic, as can interference between neighbouring qubits.
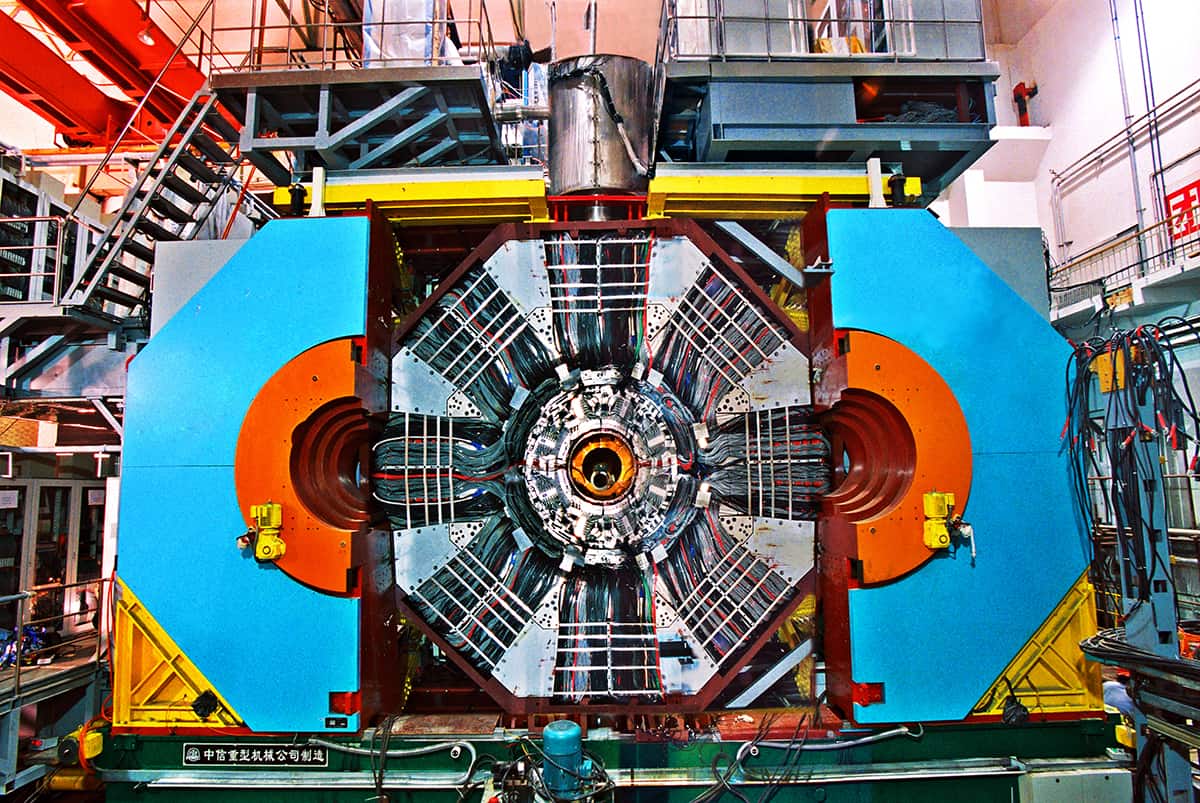
The ideal solution – a strategy called error correction – involves storing the same information across multiple qubits, such that errors will be detected and corrected when one or more of the qubits are impacted by noise. The problem with these so-called fault-tolerant quantum computers is their requirement for a large number of qubits (in the region of millions) – something that’s impossible to implement in current-generation small-scale quantum architectures.
Instead, the designers of today’s Noisy Intermediate-Scale Quantum (NISQ) computers can either accept the noise effects as they are or partially recover the errors algorithmically – i.e. without increasing the number of qubits – in a process known as error mitigation. Several algorithms are known to impart resilience against noise in small-scale quantum computers, such that “quantum advantage” may be observable in specific high-energy physics applications despite the inherent limitations of current-generation quantum computers.
One such line of enquiry at IHEP focuses on quantum simulation, applying ideas originally put forward by Richard Feynman around the use of quantum devices to simulate the time evolution of quantum systems – for example, in lattice quantum chromodynamics (QCD). For context, the Standard Model describes all the fundamental interactions among the elementary particles apart from the gravitational force – i.e. tying together the electromagnetic, weak and strong forces. In this way, the model comprises two sets of so-called quantum gauge field theories: the Glashow–Weinberg–Salam model (providing a unified description of the electromagnetic and weak forces) and QCD (for the strong forces).
It’s generally the case that the quantum gauge field theories cannot be solved analytically, with most predictions for experiments derived from continuous-improvement approximation methods (also known as perturbation). Right now, IHEP staff scientists are working on directly simulating gauge fields with quantum circuits under simplified conditions (for example, in reduced-space-time dimensions or by utilizing finite groups or other algebraic methods). Such approaches are compatible with current iterations of NISQ computers and represent foundational work for a more complete implementation of lattice QCD in the near future.
The QuIHEP quantum simulator
As an extension of its ambitious quantum R&D programme, IHEP has established QuIHEP, a quantum computing simulator platform that enables scientists and students to develop and optimize quantum algorithms for research studies in high-energy physics.
For clarity, quantum simulators are classical computing frameworks that try to emulate or “simulate” the behaviour of quantum computers. Quantum simulation, on the other hand, utilizes actual quantum computing hardware to simulate the time evolution of a quantum system – e.g. the lattice QCD studies at IHEP (see main text).
As such, QuIHEP offers a user-friendly and interactive development environment that exploits existing high-performance computing clusters to simulate up to about 40 qubits. The platform provides a composer interface for education and introduction (demonstrating, for example, how quantum circuits are constructed visually). The development environment is based on Jupyter open-source software and combined with an IHEP user authentication system.
In the near term, QuIHEP will link up with distributed quantum computing resources across China to establish a harmonized research infrastructure. The goal: to support industry-academia collaboration and education and training in quantum science and engineering.
Machine learning: the quantum way
Another quantum research theme at IHEP involves quantum machine learning, which can be grouped into four distinct approaches: CC, CQ, QC, QQ (with C – classical; Q – quantum). In each case, the first letter corresponds to the data type and the latter to the type of the computer that runs the algorithm. The CC scheme, for example, fully utilizes classical data and classical computers, though runs quantum-inspired algorithms.
The most promising use-case being pursued at IHEP, however, involves the CQ category of machine learning, where the classical data type is mapped and trained in quantum computers. The motivation here is that by exploiting the fundamentals of quantum mechanics – the large Hilbert space, superposition and entanglement – quantum computers will be able to learn more effectively from large-scale datasets to optimize the resultant machine-learning methodologies.
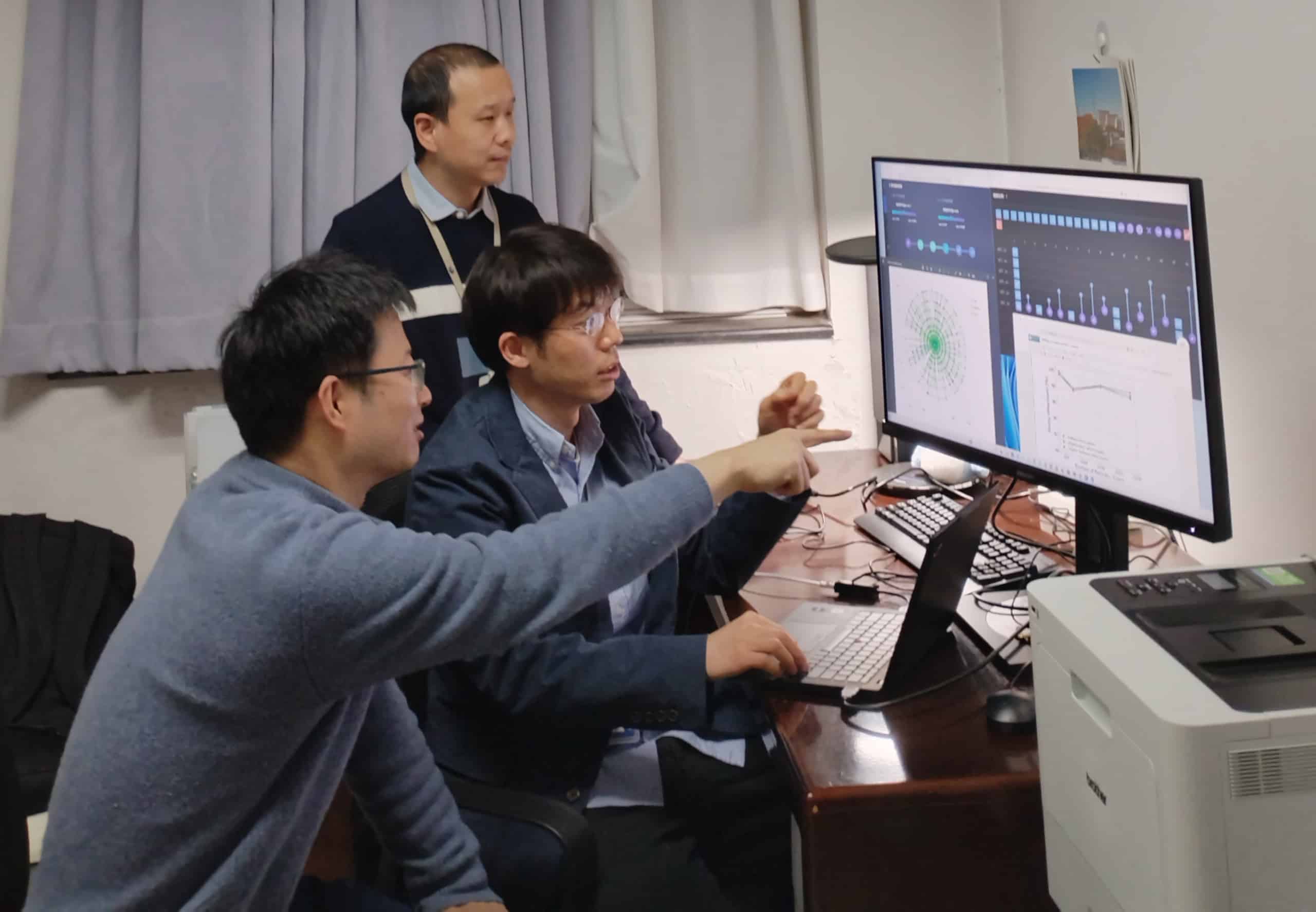
To understand the potential for quantum advantage, IHEP scientists are currently working on “rediscovering” the exotic particle Zc(3900) using quantum machine learning. In terms of the back-story: Zc(3900) is an exotic subatomic particle made up of quarks (the building blocks of protons and neutrons) and believed to be the first tetraquark state observed experimentally – an observation that, in the process, deepened our understanding of QCD. The particle was discovered in 2013 by the Beijing Spectrometer (BESIII) detector at the Beijing Electron–Positron Collider (BEPCII), with independent observation by the Belle experiment at Japan’s KEK particle physics laboratory.
QUANT-NET’s testbed innovations: reimagining the quantum network
As part of this R&D study, a team led by IHEP’s Jiaheng Zou, and including colleagues from Shandong University and the University of Jinan, deployed the so-called Quantum Support Vector Machine algorithm (a quantum variant of a classical algorithm) for the training along with simulated signals of Zc(3900) and randomly selected events from the real BESIII data as backgrounds.
Using the quantum machine-learning approach, the performance is competitive versus classical machine-learning systems – though, crucially, with a smaller training dataset and fewer data features. Investigations are ongoing to demonstrate enhanced signal sensitivity with quantum computing, work that could ultimately point the way to the discovery of new exotic particles in future experiments.
- SEO Powered Content & PR Distribution. Get Amplified Today.
- PlatoData.Network Vertical Generative Ai. Empower Yourself. Access Here.
- PlatoAiStream. Web3 Intelligence. Knowledge Amplified. Access Here.
- PlatoESG. Carbon, CleanTech, Energy, Environment, Solar, Waste Management. Access Here.
- PlatoHealth. Biotech and Clinical Trials Intelligence. Access Here.
- Source: https://physicsworld.com/a/ihep-seeks-quantum-opportunities-to-fast-track-fundamental-science/