Exclusive In July, the White House announced that seven large tech players have committed to AI safety measures, including the deployment of watermarking to ensure that algorithmically-generated content can be distinguished from the work of actual people.
Among those giants, Amazon, Google, and OpenAI have all specifically cited watermarking – techniques for adding information to text and images that attests to the provenance of the content – as one way they intend to defend against misinformation, fraud, and deepfakes produced by their generative AI models.
The goal here being that AI-generated material will be subtly marked so that it can be detected and identified as such if someone tries to pass off the content as human made.
But digital watermarking in images – adding noise when content is created and then detecting the presence of that noise pattern within image data sets – may not offer much of a safety guarantee, academics have warned.
A team at the University of Maryland in the US has looked into the reliability of watermarking techniques for digital images and found they can be defeated fairly easily. They describe their findings in a preprint paper scheduled for release this evening on ArXiv, “Robustness of AI-Image Detectors: Fundamental Limits and Practical Attacks.”
Approaches taken by Google and other tech giants to watermark the output of their generative images as a defense is not going to work
“In this work, we reveal fundamental and practical vulnerabilities of image watermarking as a defense against deepfakes,” said Soheil Feizi, associate professor of computer science at the University of Maryland, in an email to The Register.
“This shows current approaches taken by Google and other tech giants to watermark the output of their generative images as a defense is not going to work.”
The findings of the University of Maryland boffins – Mehrdad Saberi, Vinu Sankar Sadasivan, Keivan Rezaei, Aounon Kumar, Atoosa Chegini, Wenxiao Wang, and Soheil Feizi – indicate that there’s a fundamental trade-off between the evasion error rate (the percentage of watermarked images detected as unmarked – ie, false negatives) and the spoofing error rate (the percentage of unmarked images detected as watermarked – false positives).
To put that another way, watermark detection schemes can have high performance (few false negatives) or high robustness (few false positives), but not both at once.
The authors of the paper have devised an attack technique for low-perturbation images (with imperceptible watermarks) called diffusion purification that was originally proposed as a defense against adversarial examples – input that deliberately makes a model make mistakes. It involves adding Gaussian noise to images and then using the denoising process of diffusion models to eliminate the added data.
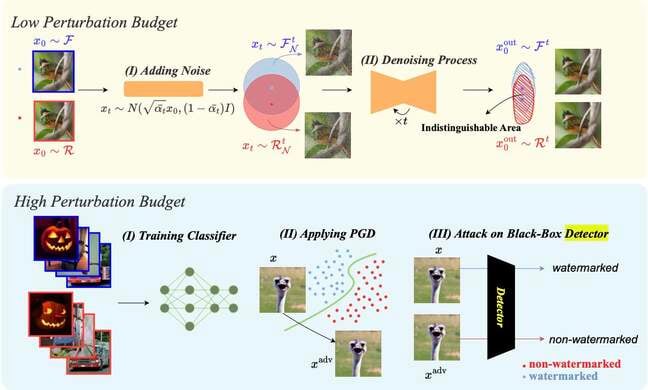
Chart from Robustness of AI-Image Detectors: Fundamental Limits and Practical Attacks … Click to enlarge
And for high-perturbation images (perceptible watermarks) that aren’t open to the diffusion purification attack, the researchers developed a spoofing mechanism that has the potential to make non-watermarked images appear to be watermarked. That scenario, the authors say, could have adverse financial or public relations consequences for firms selling AI models.
“Our [high-perturbation] attack functions by instructing watermarking models to watermark a white noise image and then blending this noisy watermarked image with non-watermarked ones to deceive the detector into flagging them as watermarked,” the paper explains.
Asked whether there are parallels in the dwindling gap between humans and machines in CAPTCHA image puzzle for detecting differences between human and machine-generated content, Feizi and Mehrdad Saberi, a doctoral student at the University of Maryland and lead author of the paper, said machine learning is becoming increasingly capable.
“Machine learning is undeniably advancing day by day, demonstrating the potential to match or even surpass human performance,” said Feizi and Saberi in an email to The Register.
“This suggests that tasks such as deciphering CAPTCHA images or generating text may already be within the capabilities of AI, rivaling human proficiency.
“In the case of generating images and videos, AI-generated content is becoming more similar to real content, and the task of distinguishing them from each other might be impossible in the near future regardless of what technique is used. In fact, we show a robustness vs. reliability tradeoff for classification-based deepfake detectors in our work.”
The Register asked Google and OpenAI to comment, and neither responded.
Feizi and Saberi said they did not specifically analyze Google or OpenAI’s watermarking mechanisms because neither company had made their watermarking source code public.
“But our attacks are able to break every existing watermark that we have encountered,” they said.
“Similar to some other problems in computer vision (eg, adversarial robustness), we believe image watermarking will be a race between defenses and attacks in the future. So while new robust watermarking methods might be proposed in the future, new attacks will also be proposed to break them.” ®
- SEO Powered Content & PR Distribution. Get Amplified Today.
- PlatoData.Network Vertical Generative Ai. Empower Yourself. Access Here.
- PlatoAiStream. Web3 Intelligence. Knowledge Amplified. Access Here.
- PlatoESG. Carbon, CleanTech, Energy, Environment, Solar, Waste Management. Access Here.
- PlatoHealth. Biotech and Clinical Trials Intelligence. Access Here.
- Source: https://go.theregister.com/feed/www.theregister.com/2023/10/02/watermarking_security_checks/